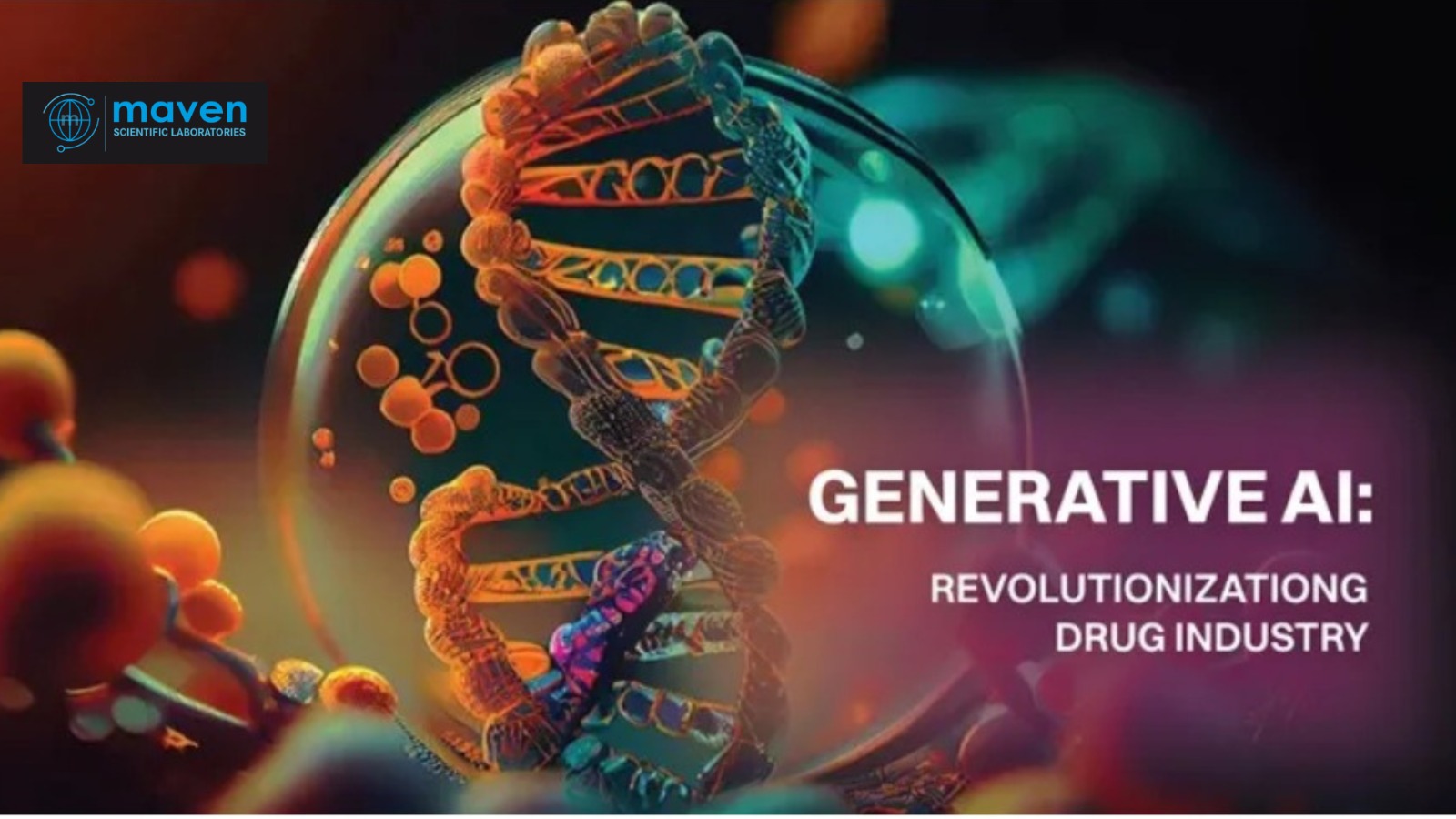
The Revolutionary Role Of Generative Models In Drug Discovery
Introduction
Drug discovery is a complex, time-consuming, and costly process that involves identifying and developing new drugs to treat various diseases. With the advent of artificial intelligence (AI) and machine learning (ML), generative models have emerged as a game-changer in the pharmaceutical industry. In this blog, we'll explore the exciting applications of generative models in drug discovery and their potential to transform the field.
What are Generative Models?
Generative models are a type of AI algorithm that can generate new, synthetic data that resembles existing data. In the context of drug discovery, generative models can produce novel molecular structures, predict their properties, and identify potential drug candidates. Most common types of generative models include:
- Generative Adversarial Networks (GANs): Consist of two neural networks, a generator, and a discriminator, that work against each other to produce realistic data.
- Variational Autoencoders (VAEs): Learn efficient representations of data and generate new samples by tweaking these representations.
- Transformer Models: Powerful models like GPT (Generative Pre-trained Transformer) and BERT have been adapted to generate complex sequences, including those that can represent chemical structures.
Applications of Generative Models in Drug Discovery
- Molecular Generation: Generative models can generate novel molecular structures with desired properties, such as binding affinity or solubility. This can lead to the discovery of new drug candidates with improved efficacy and reduced side effects.
- Property Prediction: Generative models can predict the properties of generated molecules, such as toxicity, absorption, or metabolism. This can help filter out unsuitable candidates and prioritize promising ones.
- Lead Optimization: Generative models can optimize lead compounds by generating new structures with improved properties, such as potency or selectivity.
- De Novo Design: Generative models can design novel molecules from scratch, without relying on existing scaffolds or templates.
- Virtual Screening: GANs and VAEs can simulate millions of potential interactions, identifying promising candidates faster than traditional methods. These models can generate compounds tailored to specific biological targets, increasing the chances of finding viable drugs.
- Drug Repurposing: By understanding the molecular interactions of existing drugs, generative models can suggest new therapeutic targets, expanding the drug's applicability.
- Synthesis Planning: Models can predict chemical reactions and propose efficient synthetic routes, optimizing the drug development process.
Benefits of Generative Models in Drug Discovery
- Speed: Generative models can generate thousands of novel molecules in a matter of minutes, accelerating the drug discovery process.
- Innovation: Generative models can explore new chemical spaces and generate novel structures that human chemists may not have considered.
- Cost-Effectiveness: Generative models can reduce the need for physical synthesis and testing, saving time and resources.
- Personalized Medicine: Generative models can design drugs tailored to individual patients' needs, revolutionizing personalized medicine.
Challenges and Future Directions
- Data Quality: High-quality training data is essential for generative models to produce accurate and reliable results.
- Interpretability: Understanding how generative models make predictions and generate molecules is crucial for building trust and improving performance.
- Integration: Integrating generative models with existing drug discovery workflows and tools is essential for seamless adoption.
Conclusion
Generative models have the potential to revolutionize drug discovery by accelerating the identification of novel drug candidates, improving their properties, and reducing costs. As the field continues to evolve, we can expect to see more innovative applications of generative models in drug discovery, leading to better treatments and improved patient outcomes.
References
- Sanchez-Lengeling, B., & Aspuru-Guzik, A. (2018). Inverse molecular design using machine learning: Generative models for matter engineering. Science, 361(6400), 360-365.
- Gómez-Bombarelli, R., et al. (2018). Automatic chemical design using a data-driven continuous representation of molecules. ACS Central Science, 4(2), 268-276.
- Zhavoronkov, A., et al. (2019). Deep learning enables rapid identification of potent DDR1 kinase inhibitors. Nature Biotechnology, 37(9), 1038-1044.